资源简介
code.zip
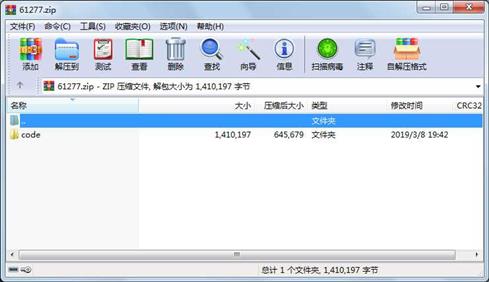
代码片段和文件信息
import numpy as np
import tensorflow as tf
import random
from collections import Counter
from nltk.tokenize import word_tokenize
“““
‘I‘m super man‘
tokenize:
[‘I‘ ‘‘m‘ ‘super‘‘man‘ ]
“““
from nltk.stem import WordNetLemmatizer
“““
词形还原(lemmatizer),即把一个任何形式的英语单词还原到一般形式,与词根还原不同(stemmer),后者是抽取一个单词的词根。
“““
pos_file = ‘pos.txt‘
neg_file = ‘neg.txt‘
# 创建词汇表
def create_lexicon(pos_file neg_file):
lex = []
# 读取文件
def process_file(f):
with open(pos_file ‘r‘errors=‘ignore‘) as f:
lex = []
lines = f.readlines()
for line in lines:
words = word_tokenize(line.lower())
lex += words
return lex
lex += process_file(pos_file)
lex += process_file(neg_file)
lemmatizer = WordNetLemmatizer()
lex = [lemmatizer.lemmatize(word) for word in lex] # 词形还原 (cats->cat)
word_count = Counter(lex)
#print(word_count)
# {‘.‘: 13944 ‘‘: 10536 ‘the‘: 10120 ‘a‘: 9444 ‘and‘: 7108 ‘of‘: 6624 ‘it‘: 4748 ‘to‘: 3940......}
# 去掉一些常用词像thea and等等,和一些不常用词; 这些词对判断一个评论是正面还是负面没有做任何贡献
lex = []
for word in word_count:
if word_count[word] < 1000 and word_count[word] > 9: # 这写死了,好像能用百分比
lex.append(word) # 齐普夫定律-使用Python验证文本的Zipf分布 http://blog.topspeedsnail.com/archives/9546
return lex
lex = create_lexicon(pos_file neg_file)
#lex里保存了文本中出现过的单词。
# 把每条评论转换为向量 转换原理:
# 假设lex为[‘woman‘ ‘great‘ ‘feel‘ ‘actually‘ ‘looking‘ ‘latest‘ ‘seen‘ ‘is‘] 当然实际上要大的多
# 评论‘i think this movie is great‘ 转换为 [01000001] 把评论中出现的字在lex中标记,出现过的标记为1,其余标记为0
def normalize_dataset(lex):
dataset = []
# lex:词汇表;review:评论;clf:评论对应的分类,[01]代表负面评论 [10]代表正面评论
def string_to_vector(lex review clf):
words = word_tokenize(line.lower())
lemmatizer = WordNetLemmatizer()
words = [lemmatizer.lemmatize(word) for word in words]
features = np.zeros(len(lex))
for word in words:
if word in lex:
features[lex.index(word)] = 1 # 一个句子中某个词可能出现两次可以用+=1,其实区别不大
return [features clf]
with open(pos_file ‘r‘errors=‘ignore‘) as f:
lines = f.readlines()
for line in lines:
one_sample = string_to_vector(lex line [10]) # [array([ 0. 1. 0. ... 0. 0. 0.]) [10]]
dataset.append(one_sample)
with open(neg_file ‘r‘errors=‘ignore‘) as f:
lines = f.readlines()
for line in lines:
one_sample = string_to_vector(lex line [01]) # [array([ 0. 0. 0. ... 0. 0. 0.]) [01]]]
dataset.append(one_sample)
#print(len(dataset))
return dataset
dataset = normalize_dataset(lex)
random.shuffle(dataset)
“““
到此完成了数据的整理工作
“““
# 取样本中的10%做为测试数据
test_size = int(len(dataset) * 0.1)
dataset = np.array(dataset) # 列表转换为矩阵
train_dataset = dataset[:-test_size]
test_dataset = dataset[-test_size:]
# Feed-Forward Neural Network
# 定义每个层有多少‘神经元‘‘
n_input_layer = len(lex) # 输入层
n_layer_1 = 1000 # hide layer1
n_layer_2 = 1000 # hide layer2
n_output_layer = 2 # 输出层
# 定义待训练的神经网络
def neural_network
属性 大小 日期 时间 名称
----------- --------- ---------- ----- ----
目录 0 2019-03-08 19:42 code\
文件 6214 2019-03-08 19:39 code\comment_classification.py
文件 612846 2018-02-08 22:50 code\neg.txt
文件 626757 2018-02-08 22:50 code\pos.txt
文件 164380 2019-03-08 19:41 code\注意事项.docx
- 上一篇:鼠标跟随星星特效
- 下一篇:51单片机18B20
相关资源
- 价值2k的H漫画小说系统
- Pythonamp;课堂amp;笔记(高淇amp;400;集第
- ddos压力测试工具99657
- UML建模大全
- 开源1A锂电池充电板TP4056原理图+PCB
- m1卡 ic卡可选择扇区初始化加密软件
- TSCC.exe
- FTP课程设计(服务端+客户端)
- 计算机图形学 边填充算法实现代码
- 电力系统潮流计算程序集合
- oracle数据迁移项目实施方案
- Web Api 通过文件流 文件到本地
- Visio图标-最新最全的网络通信图标库
- Spire API文档
- OpenGL参考手册
- Python中Numpy库最新教程
- SPD博士V5.3.exe
- 直流无刷电机方波驱动 stm32 例程代码
- layui后台管理模板
- 仿知乎界面小程序源代码
- 云平台-阿里云详细介绍
- photoshop经典1000例
- scratch垃圾分类源码(最终版本).sb
- IAR ARM 7.8破解
- TI CCS V5.4 安装步骤及破解文件
- 松下plc FP-XH的驱动
- 局域网硬件信息收集工具
- 加快Windows XP操作系统开机速度
- 联想启天M4350 BIOS升级文件
- 操作系统教程课后答案华中科技大学
评论
共有 条评论