资源简介
回声状态网络(ESN)以及确定性跳跃循环状态网络(CRJ)的实现
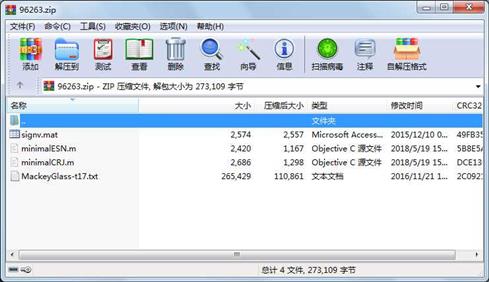
代码片段和文件信息
% A minimalistic Echo State Networks demo with Mackey-Glass (delay 17) data
% in “plain“ Matlab.
% by Mantas Lukosevicius 2012
% http://minds.jacobs-university.de/mantas
% load the data
trainLen = 3000;
testLen = 1000;
initLen = 100;
data = load(‘MackeyGlass-t17.txt‘);
load(‘signv.mat‘);
% plot some of it
% figure(10);
% plot(data(1:1000));
% title(‘A sample of data‘);
% generate the ESN reservoir
inSize = 1; outSize = 1;
resSize = 500;
a = 0.3; % leaking rate
signal = y(1:5001:2); %signal of W_in
%rand( ‘seed‘ 42 );
Win = (zeros(resSize1+inSize)+0.5) .* signal; %r=0.5
W = eye(resSize) * 0.8;
W = circshift(W[12]); % generate circle
W = reshape(W1resSize*resSize);
step = 10;
for i=1:resSize
if mod(i step) == 1
W(i) = 0.7;
end
end
W = reshape(WresSizeresSize)
% Option 1 - direct scaling (quick&dirty reservoir-specific):
% W = W .* 0.13;
% Option 2 - normalizing and setting spectral radius (correct slower):
disp ‘Computing spectral radius...‘;
opt.disp = 0;
rhoW = abs(eigs(W1‘LM‘opt));
disp ‘done.‘
W = W .* ( 1.25 /rhoW);
% allocated memory for the design (collected states) matrix
X = zeros(1+inSize+resSizetrainLen-initLen);
% set the corresponding target matrix directly
Yt = data(initLen+2:trainLen+1)‘;
% run the reservoir with the data and collect X
x = zeros(resSize1);
for t = 1:trainLen
u = data(t);
x = (1-a)*x + a*tanh( Win*[1;u] + W*x );
if t > initLen
X(:t-initLen) = [1;u;x];
end
end
% train the output
reg = 1e-8; % regularization coefficient
X_T = X‘;
% Wout = Yt*X_T * inv(X*X_T + reg*eye(1+inSize+resSize));
Wout = Yt*X_T / (X*X_T + reg*eye(1+inSize+resSize));
% Wout = Yt*pinv(X);
% run the trained ESN in a generative mode. no need to initialize here
% because x is initialized with training data and we continue from there.
Y = zeros(outSizetestLen);
u = data(trainLen+1);
for t = 1:testLen
x = (1-a)*x + a*tanh( Win*[1;u] + W*x );
y = Wout*[1;u;x];
Y(:t) = y;
% generative mode:
u = y;
% this would be a predictive mode:
u = data(trainLen+t+1);
end
errorLen = 1000;
mse = sum((data(trainLen+2:trainLen+errorLen+1)‘-Y(11:errorLen)).^2)./errorLen;
disp( [‘MSE = ‘ num2str( mse )] );
% plot some signals
figure(1);
plot( data(trainLen+2:trainLen+testLen+1) ‘color‘ [00.750] );
hold on;
plot( Y‘ ‘b‘ );
hold off;
axis tight;
title(‘Target and generated signals y(n) starting at n=0‘);
legend(‘Target signal‘ ‘Free-running predicted signal‘);
figure(2);
plot( X(1:201:200)‘ );
title(‘Some reservoir activations x(n)‘);
figure(3);
bar( Wout‘ )
title(‘Output weights W^{out}‘);
属性 大小 日期 时间 名称
----------- --------- ---------- ----- ----
文件 2686 2018-05-19 15:27 minimalCRJ.m
文件 2420 2018-05-19 15:00 minimalESN.m
文件 2574 2015-12-10 00:33 signv.mat
文件 265429 2016-11-21 18:13 MackeyGlass-t17.txt
相关资源
- res10_300x300_ssd_iter_140000.caffemodel与dep
- 推箱子及人工智能寻路C 源代码
- 北航人工智能原理课大作业源代码,
- AI人工智能学习资料全套
- 用户网络行为画像 大数据中的用户网
- 中科院自动化所历年模式识别博士题
- 华南理工大学人工智能期末考试卷
- LabVIEW实现Fuzzy_PID的补充资源
- 微信小程序Demo/---欧拉蜜自然语言理解
- 微信小程序完整Demo--支持人工智能对
- 艾媒-2017年中国人工智能产业专题研究
- 工信部人工智能产业人才岗位能力标
- AMiner:2018年人工智能之自动驾驶研究
- 艾瑞咨询:2018年中国人工智能+金融行
- 2019技术趋势:人工智能报告
- 法院行业方案宣讲稿-海康
- 8.2 心智探奇 人类心智的起源与进化
- 人工智能尼尔森,2003,第1版
- 乌镇指数全球人工智能发展报告2017投
- 模式识别之特征选择
- springMVC的学习代码
- 人工智能全部课件和作业题
- 哥德尔、艾舍尔、巴赫——集异璧之
- 西电人工智能课件
- 面试题答案-40万年薪岗位面试到底问
- 旺宝创业计划书
- 人工智能综述
- 人工智能领域顶会AAAI 2018 论文列表
- AI 全套教学视频三
- 人工智能初步学习总结
评论
共有 条评论