资源简介
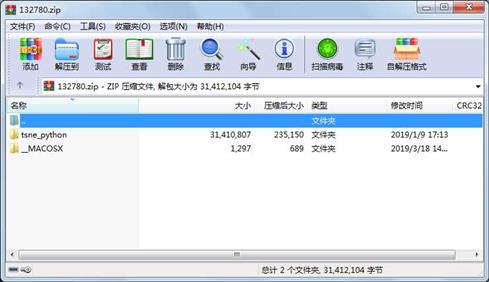
代码片段和文件信息
#
# tsne.py
#
# Implementation of t-SNE in Python. The implementation was tested on Python
# 2.7.10 and it requires a working installation of NumPy. The implementation
# comes with an example on the MNIST dataset. In order to plot the
# results of this example a working installation of matplotlib is required.
#
# The example can be run by executing: ‘ipython tsne.py‘
#
#
# Created by Laurens van der Maaten on 20-12-08.
# Copyright (c) 2008 Tilburg University. All rights reserved.
import numpy as np
import pylab
def Hbeta(D=np.array([]) beta=1.0):
“““
Compute the perplexity and the P-row for a specific value of the
precision of a Gaussian distribution.
“““
# Compute P-row and corresponding perplexity
P = np.exp(-D.copy() * beta)
sumP = sum(P)
H = np.log(sumP) + beta * np.sum(D * P) / sumP
P = P / sumP
return H P
def x2p(X=np.array([]) tol=1e-5 perplexity=30.0):
“““
Performs a binary search to get P-values in such a way that each
conditional Gaussian has the same perplexity.
“““
# Initialize some variables
print(“Computing pairwise distances...“)
(n d) = X.shape
sum_X = np.sum(np.square(X) 1)
D = np.add(np.add(-2 * np.dot(X X.T) sum_X).T sum_X)
P = np.zeros((n n))
beta = np.ones((n 1))
logU = np.log(perplexity)
# Loop over all datapoints
for i in range(n):
# Print progress
if i % 500 == 0:
print(“Computing P-values for point %d of %d...“ % (i n))
# Compute the Gaussian kernel and entropy for the current precision
betamin = -np.inf
betamax = np.inf
Di = D[i np.concatenate((np.r_[0:i] np.r_[i+1:n]))]
(H thisP) = Hbeta(Di beta[i])
# Evaluate whether the perplexity is within tolerance
Hdiff = H - logU
tries = 0
while np.abs(Hdiff) > tol and tries < 50:
# If not increase or decrease precision
if Hdiff > 0:
betamin = beta[i].copy()
if betamax == np.inf or betamax == -np.inf:
beta[i] = beta[i] * 2.
else:
beta[i] = (beta[i] + betamax) / 2.
else:
betamax = beta[i].copy()
if betamin == np.inf or betamin == -np.inf:
beta[i] = beta[i] / 2.
else:
beta[i] = (beta[i] + betamin) / 2.
# Recompute the values
(H thisP) = Hbeta(Di beta[i])
Hdiff = H - logU
tries += 1
# Set the final row of P
P[i np.concatenate((np.r_[0:i] np.r_[i+1:n]))] = thisP
# Return final P-matrix
print(“Mean value of sigma: %f“ % np.mean(np.sqrt(1 / beta)))
return P
def pca(X=np.array([]) no_dims=50):
“““
Runs PCA on the NxD array X in order to reduce its dimensionality to
no_dims dimensions.
“““
print(“Preprocessing the data using P
属性 大小 日期 时间 名称
----------- --------- ---------- ----- ----
目录 0 2019-01-09 17:13 tsne_python\
文件 42500 2008-12-30 18:13 tsne_python\mnist2500_labels.txt
目录 0 2019-03-18 14:46 __MACOSX\
目录 0 2019-03-18 14:46 __MACOSX\tsne_python\
文件 268 2008-12-30 18:13 __MACOSX\tsne_python\._mnist2500_labels.txt
文件 31362500 2008-12-30 18:13 tsne_python\mnist2500_X.txt
文件 268 2008-12-30 18:13 __MACOSX\tsne_python\._mnist2500_X.txt
文件 5807 2017-12-15 22:27 tsne_python\tsne.py
文件 549 2017-12-15 22:27 __MACOSX\tsne_python\._tsne.py
文件 212 2019-01-09 17:13 __MACOSX\._tsne_python
- 上一篇:Epicor10中Adapter使用封装
- 下一篇:基于dsp的胎心率测量
相关资源
- 推箱子及人工智能寻路C 源代码
- 北航人工智能原理课大作业源代码,
- AI人工智能学习资料全套
- 用户网络行为画像 大数据中的用户网
- 中科院自动化所历年模式识别博士题
- 华南理工大学人工智能期末考试卷
- LabVIEW实现Fuzzy_PID的补充资源
- 微信小程序Demo/---欧拉蜜自然语言理解
- 微信小程序完整Demo--支持人工智能对
- 艾媒-2017年中国人工智能产业专题研究
- 工信部人工智能产业人才岗位能力标
- AMiner:2018年人工智能之自动驾驶研究
- 艾瑞咨询:2018年中国人工智能+金融行
- 2019技术趋势:人工智能报告
- 法院行业方案宣讲稿-海康
- 8.2 心智探奇 人类心智的起源与进化
- 人工智能尼尔森,2003,第1版
- 乌镇指数全球人工智能发展报告2017投
- 模式识别之特征选择
- springMVC的学习代码
- 人工智能全部课件和作业题
- 哥德尔、艾舍尔、巴赫——集异璧之
- 西电人工智能课件
- 面试题答案-40万年薪岗位面试到底问
- 旺宝创业计划书
- 人工智能综述
- 人工智能领域顶会AAAI 2018 论文列表
- AI 全套教学视频三
- 人工智能初步学习总结
- stm32实现的五子棋AI人机对战+人人对战
评论
共有 条评论